The Mekong River Delta is a very significant rice-producing region of the world. Known as the 'Rice Bowl', this region produces more than half of Vietnam's rice crop, the country being the world's 5th largest rice producer and the 3rd largest rice exporter at the time of writing this article.
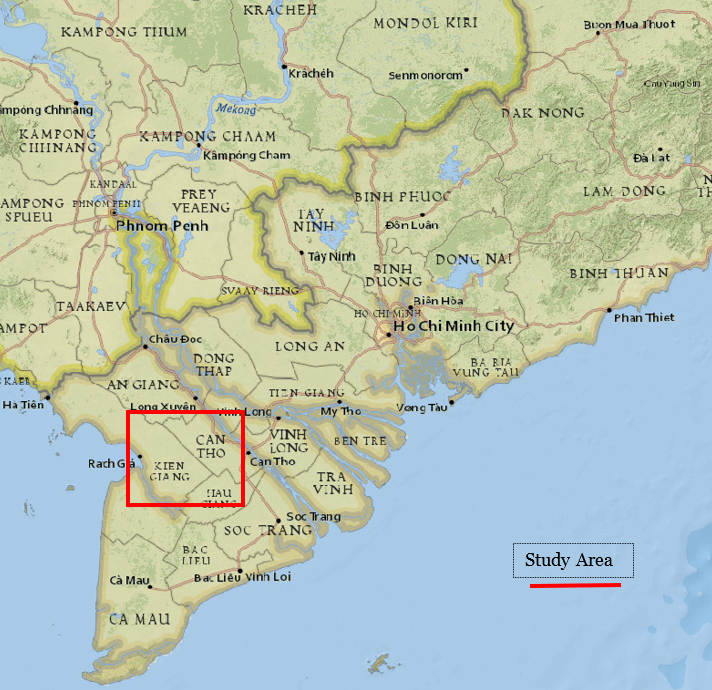
Mapping agricultural produce, especially for a staple food such as rice, is very important. Not only does it employ a significant number of farmers (over 15 million rice farmers in Vietnam itself), but also the information on its growing patterns & productivity and health impacts planners, decision makers and marketers alike.
Just this year, due to limited internal supplies, Vietnam was forced to import rice from its trading rival - my country India.
Majority of my previous work on aerial imagery involved analyzing a single satellite image and drawing conclusions out of it. Occasionally, I compare the 'output' of analysis of two images to demonstrate the difference for better understanding.
For this application, we will use Remote Sensing (15 Sentinel-1 Synthetic Aperture Radar Satellite images from April to December 2020) & knowledge of planting characteristics to detect the presence of Rice Fields in the Mekong River Valley Delta of Vietnam.
(Much thanks to RUS Copernicus for supplying the tutorial and resources to perform this analysis)
Why do we need multiple images for our analysis? That too for mapping rice fields, you may wonder. Consider the Optical imagery below -
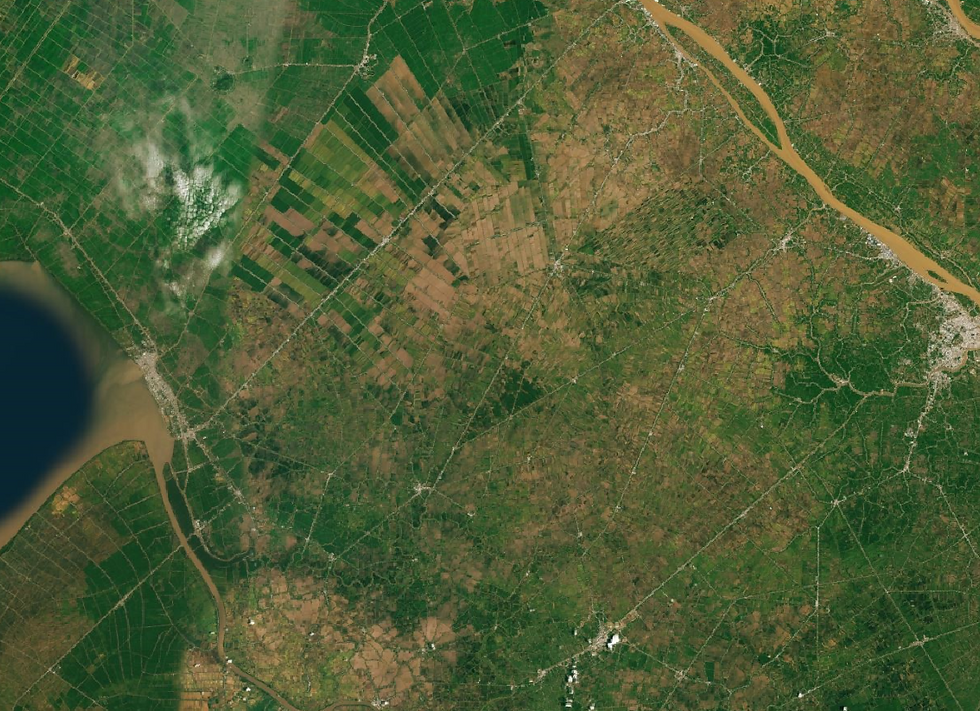
Will you be able to identify whether the dark green areas are rice fields and the brown or light green areas are not rice fields at all? Upon giving due thought, most of us would likely respond with a negative (unless you're a farmer practicing in this region).
It is difficult to deduce even if I were to give you an added piece of information - 'the image was taken during the rice harvesting season'. After all, how will you be able to tell whether a particular field has been harvested for its rice or is pending to be harvested for its rice or is barren or has been harvested for some other crop whose harvesting season coincides with that of rice.
That being said, the accuracy of your deduction may improve if I were to give you multiple images from the harvesting season, however, it still wouldn't be reasonably satisfactory / accurate.
For this application, analyzing multiple satellite radar images helps to address the problem statement. While Radar imagery has several intrinsic benefits over optical images, from this use case point of view, its main benefit lies in how the radar waves (SAR - Synthetic Aperture Radar) interact with the structural properties of the land cover, especially that of rice fields / paddy.
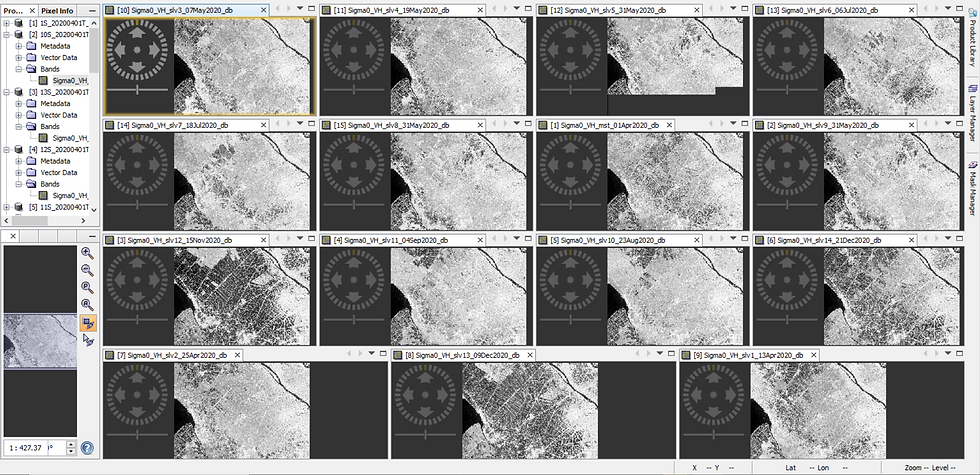

You may be aware that rice fields are inundated i.e. under water during the planting / initial stage. Only during the growth stage does the rice plant emerge from the water and becomes visible.
As you will see later, it is this very structural property which helps us to distinguish a rice field from other agricultural fields or other types of land cover in general.

When a SAR radar wave hits a flooded area the reflection (backscatter) bounces away whereas when it hits vegetation, the reflection bounces back / towards the satellite.
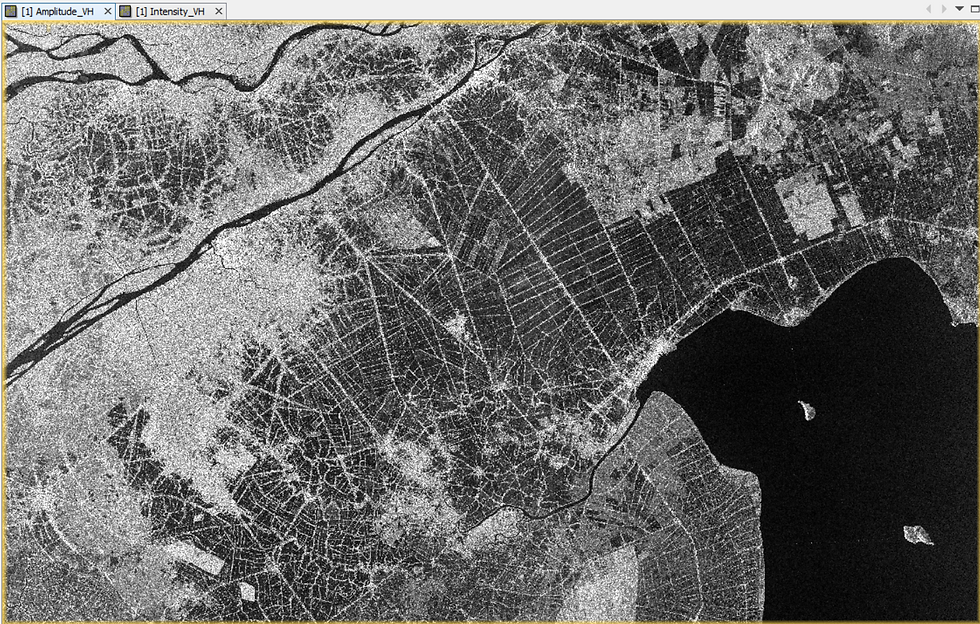
In this single, raw SAR image from 9th December, 2020 above - the darker regions represent areas with low backscatter i.e. these are likely to be inundated whereas the brighter regions represent areas with high backscatter i.e. these are likely to be vegetated.

Several processing steps are needed before the raw image is rendered to be useful for our desired application. Without delving into the technical aspects, you can visibly conclude that the post-processed image above has an enhanced B/W color diversity, has been subsetted to our study area, and is accurately geo-positioned when compared to the raw image.
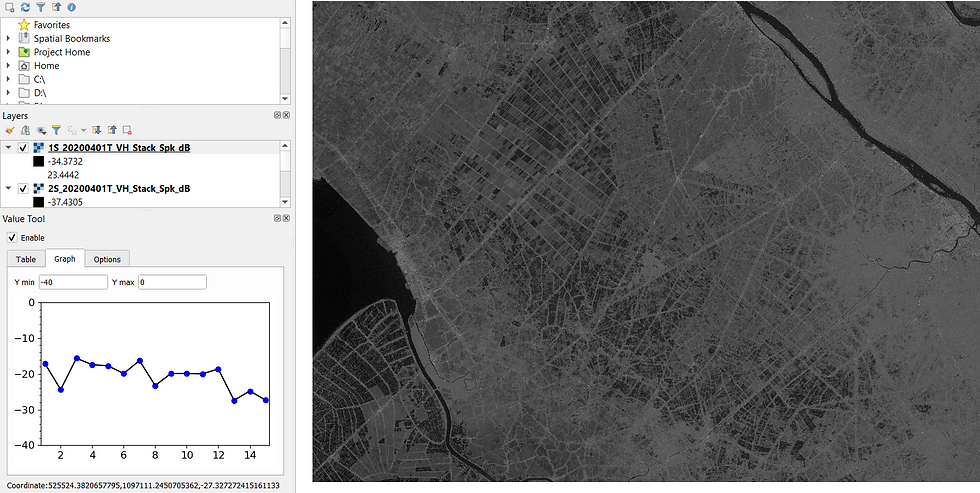
Next, we create a temporal backscatter profile i.e. load all the 15 images together and see how a particular pixel (the coordinates of which are mentioned on the bottom left of the image above) has evolved over time.
From the graph on the left, you can observe that there are three distinctive troughs i.e. pixel values with low backscatter - in the 2nd image, in the 8th image and in the 13th image respectively. These correspond to images captured on 13th April, 18th July and 15th November 2020 respectively.
You can also observe that the troughs are usually followed with periods of increasing backscatter. In Vietnam, rice is generally cultivated thrice in a year - in spring, autumn and winter. Hence, we are in a position to take a reasonable guess - that the pixel under review is used to cultivate rice i.e. is a paddy field.
We now proceed to enhance and emphasize this pixel property in a visually meaningful manner.
Which area is likely to be a rice field? It is fair to deduce that the areas which would have the highest variation in pixel backscatter values over the period of nine months are likely to be rice fields.
This is exactly what we proceed to do. From the 15 images, we will now compute a) the maximum backscatter value of the pixels, b) compute the minimum backscatter value of the pixels and compute the c) difference (max - min) backscatter value of the pixels. These 3 bands of information are used to create a single false-color composition / visual raster image which is displayed below -

From a scale of dark violet to bright blue - the dark violet parts of the map represent areas with highest backscatter variation whereas the bright blues represent areas with the lowest backscatter variation over the course of the 15 images. Areas with high backscatter difference i.e. violet are likely to be rice / paddy fields.
Please note, this is just a visual representation of the land cover and not a classification. We don't really know whether these are indeed rice fields or not but are making a reasonable guess basis the particular structural property of a rice field vis-à-vis those of other land cover types. To validate our visualization and turn it into a classification - we can use optical imagery and in-situ, supervised observations to complement / enhance the findings obtained from analyzing the radar imagery.
Hope you enjoyed this study on Remote Sensing Rice. Which crop would you suggest next?
ABOUT US
Intelloc Mapping Services | Mapmyops.com is based in Kolkata, India and engages in providing Mapping solutions that can be integrated with Operations Planning, Design and Audit workflows. These include but are not limited to - Drone Services, Subsurface Mapping Services, Location Analytics & App Development, Supply Chain Services & Remote Sensing Services. The services can be rendered pan-India, some even globally, and will aid an organization to meet its stated objectives especially pertaining to Operational Excellence, Cost Reduction, Sustainability and Growth.
Broadly, our area of expertise can be split into two categories - Geographic Mapping and Operations Mapping. The Infographic below highlights our capabilities.
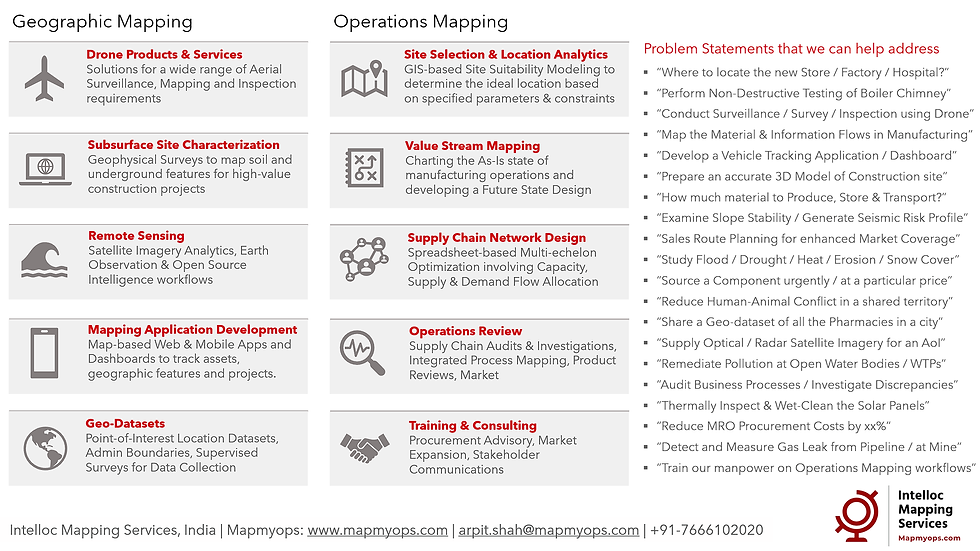
Our 'Mapping for Operations'-themed workflow demonstrations can be accessed from the firm's Website / YouTube Channel and an overview can be obtained from this flyer. Happy to address queries and respond to documented requirements. Custom Demonstration, Training & Trials are facilitated only on a paid-basis. Looking forward to being of service.
Regards,